There have been some very interesting engineering developments in both robotics and artificial intelligence (AI) recently. These new designs clearly show what I consider to be the main theme of these subjects, a convergence of the artificial and organic as engineers learn how to copy the abilities of living creatures, taking advantage of the strengths of biological systems in order to improve the functioning of their designs.
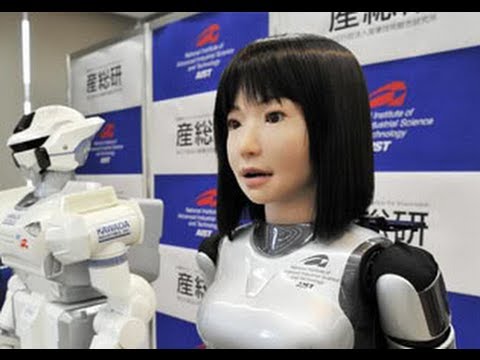
We are all aware of how awkward and clumsy the movements of robots appear when compared to the grace and dexterity of living creatures. The mechanical walk of a robot as depicted in SF movies of the 50s and 60s may be a cliché, but nevertheless it’s still pretty much true. Because of this inflexibility robots are usually designed for a single, repetitive task. Multi-tasking for robots is usually just out of the question.

With that in mind I’ll start today’s post by describing some of the work of Doctor Fumiya Iida of the Department of Engineering at the University of Cambridge in the UK. Throughout his 20-year career Dr. Iida has studied the anatomy of living creatures in an effort to improve the agility of his own robotic creations.
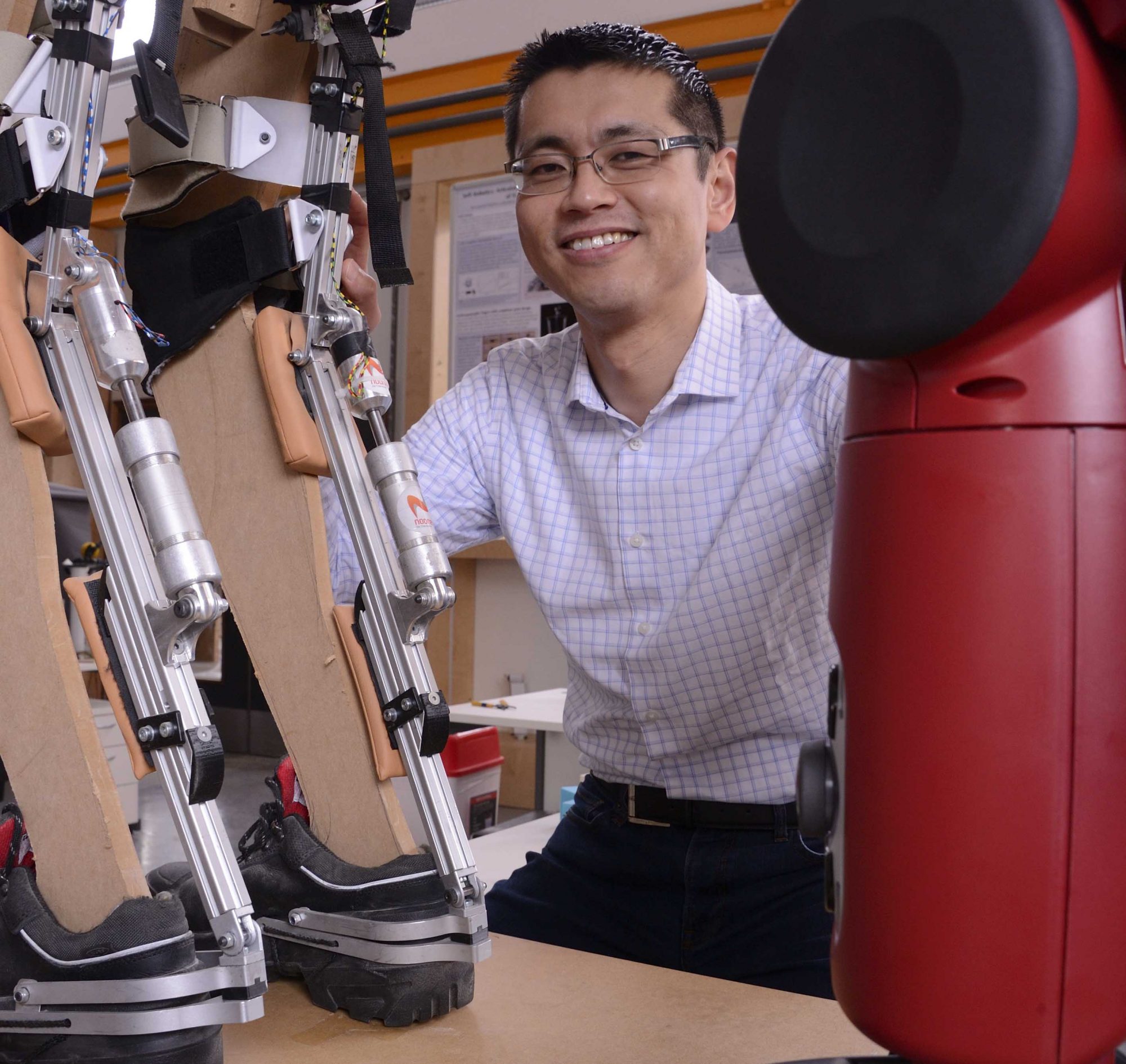
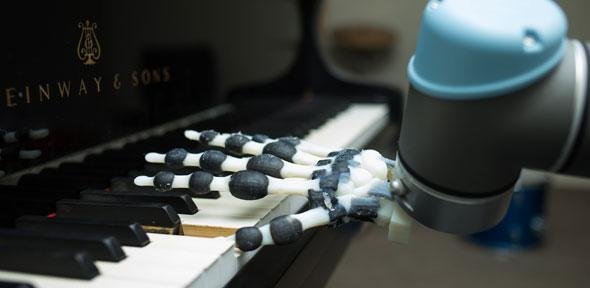
Dr. Iida has found inspiration from a wide range of different anatomical structures. Everything from the prehensile tail of a monkey to the sucker mouth of a leech can become for him a new way for a robot to move and manipulate objects. Dr. Iida and his colleagues refer to this program as ‘Bio Inspired Robotics’. Dr. Iida’s latest success has been the demonstration of a robot that can perform a labourious and backbreaking job that before now could only be accomplished by humans, picking lettuce.

Now at first you might think that picking lettuce would be an easy job to design a robot to handle. After all lettuce heads are all planted evenly spaced in straight rows. All a robotic picker has to do is go along the rows and grab the lettuce heads.
It’s not that simple, first of all a lettuce head is fairly soft and every individual head of lettuce is a somewhat different size and shape. This makes picking the lettuce heads difficult for most robots, resulting in a considerable amount of damage to the lettuce. Also, the outermost leafs of a lettuce head are generally so dirty or damaged that they have to be removed, a task that hitherto no robot has been able to carry out reliably.
Putting all that he’s learned into the problem Dr. Iida utilized a combination of visual sensors, soft grippers and a pneumatically activated knife for his robot picker. First the robot uses its cameras to locate a lettuce head before positioning itself directly above it. Then lowering itself onto the lettuce the robot pushes the unwanted leaves down and out of the way before cutting the head at its base. The robot’s soft grippers then lift the head up and place it in a basket.
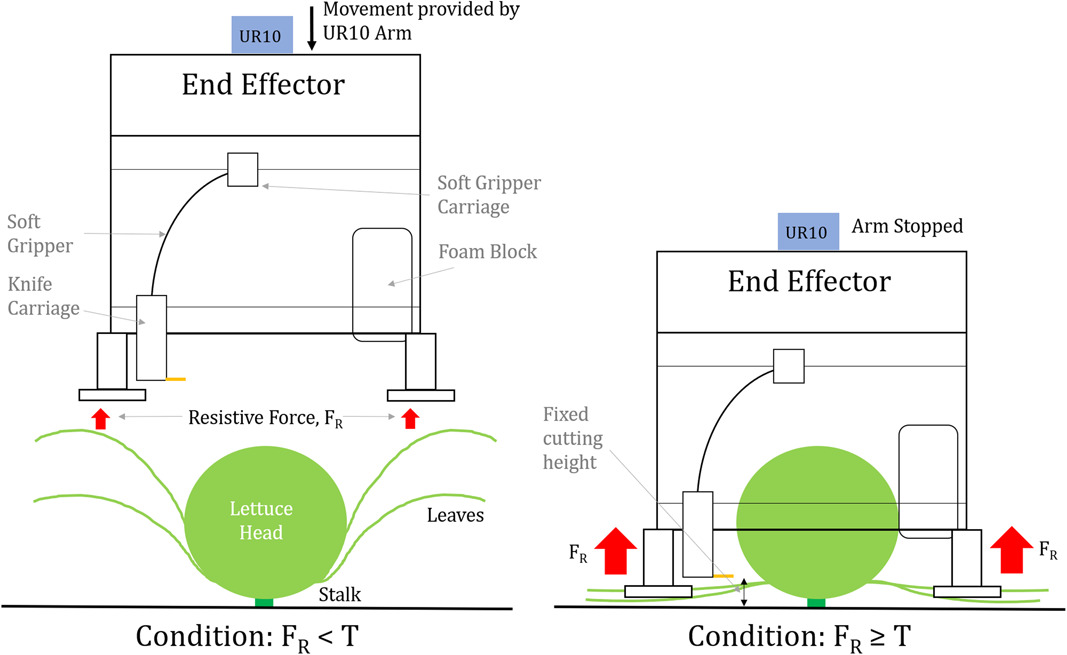

So far Dr. Iida’s robot has been able to achieve an 88% harvest success rate, good but it still needs improvement before it can replace human pickers. Nevertheless when perfected this technology could be adapted to other types of produce, finally automating what has remained one of the hardest and lowest paying of all jobs.
So, if engineers are starting to construct robots to harvest our vegetables for us, what other boring repetitive jobs can they be built to take off our hands? Well researchers at the Massachusetts Institute of Technology (MIT) are actually developing robots that can learn to do common household chores like setting the table by watching us do it!
The technology has been given the name ‘Planning with Uncertain Specifications’ or PUnS and the idea is to enable robots to perform human like planning based on observations rather than simply carrying out a list of instructions. By watching humans completing a task, like setting a table, the robot learns the goal of the task and a general idea of how to accomplish that goal. Known as ‘Linear Temporal Logic’ or LTL these computer generated formulas serve as templates for the robot to follow in order to accomplish its goal.
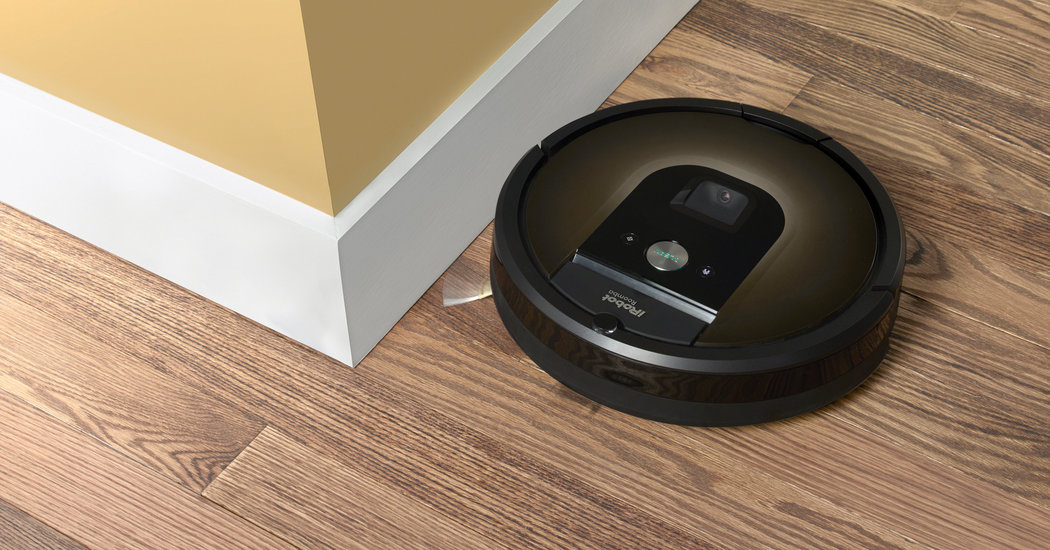
In the study the PUnS robot observed 40 humans carry out the task of setting a table and from those observations generated 25 LTL formulas for how to complete the task. At the same time the computer assigned to each formula a different confidence of success value. Starting with the highest value formula the robot was then ordered to attempt to complete the task and based on its performance it is either rewarded or punished.

In a series of 20,000 tests starting with different initial conditions the robot only made a mistake 6 times. In one test for example, the fork is hidden at the start of a test. Despite not having all of the items required to completely set the table the robot went ahead and set the rest of the dinnerware correctly. Then, when the fork was reveled the robot picked it up and placed it in the correct position, completing the task. This degree of flexibility in an automated system is unprecedented and points the way to robots learning how to accomplish different jobs not by mindlessly following a long list of instructions, in other words a program, but rather the same way humans do, by watching someone else do it. So, robots are now being designed to move more like a living creature does, and computers are being programmed to learn more like a human does. It took evolution billions of years to give living creatures those abilities but by observing and copying biological systems our robots and computers are quickly catching up. Who knows where they’ll be in another few decades.